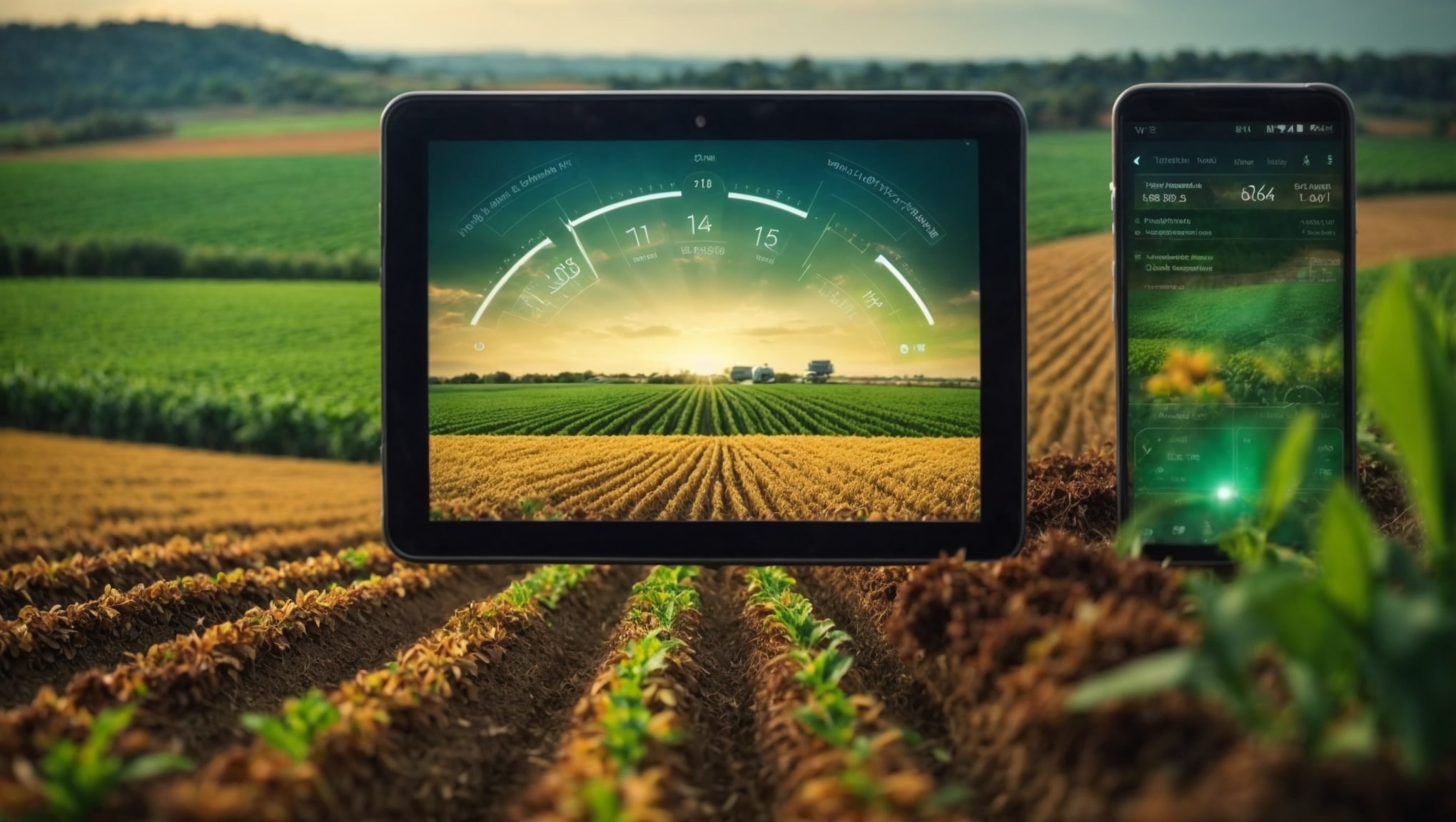
Precision Agriculture: The Present, the Future
Share



Many definitions for Precision Agriculture exist today. But the actual definition was pioneered by the USA House of Representatives in 1997 as follows: ”an integrated information- and production-based farming system that is designed to increase long term, site-specific and whole farm production efficiency, productivity and profitability while minimizing unintended impacts on wildlife and the environment”. PA simply makes the farming experience unique for every farm, based on the information collected. While the concept of merging the Information Age with traditional agriculture may not be new, it is the presence of new technologies that are redefining the whole idea. PA customizes field management into smaller areas, maximizing on data collection.
PA achieves its goals by making the most of sensors, robotic automations and digital data. It is the most important sector if food security is to be addressed.The availability of data being collected from the sensor and robotics makes the possibility of introducing Artificial Intelligence (AI) into PA a reality.
Among the many fields of AI with potential in agriculture, is image processing, a modern Deep Learning technique that has recently started to be embraced. When successfully applied, the smart farming model will address key challenges such as productivity, sustainability and food security with certainty. At the core of image processing are convolutional neural networks (CNNs) leading the way. Today, there are many applications of CNNs in agriculture and specific to this research work, related to plant and livestock disease detection.
It is well documented that pests and diseases amount a range of 20-40% of the global food waste. This basically underlines the importance of the focus topic. In agriculture, proper implementation of image processing will result in fast and accurate detection of plant diseases and livestock infections. Coupled with the availability of RGB images, CNNs are easily applicable.
Highlight
Precision Agriculture is the foundation of a food-sufficient world today and tomorrow.
“PA achieves its goals by making the most of sensors, robotic automations and digital data. It is the most important sector if food security is to be addressed.The availability of data being collected from the sensor and robotics makes the possibility of introducing Artificial Intelligence (AI) into PA a reality. ”
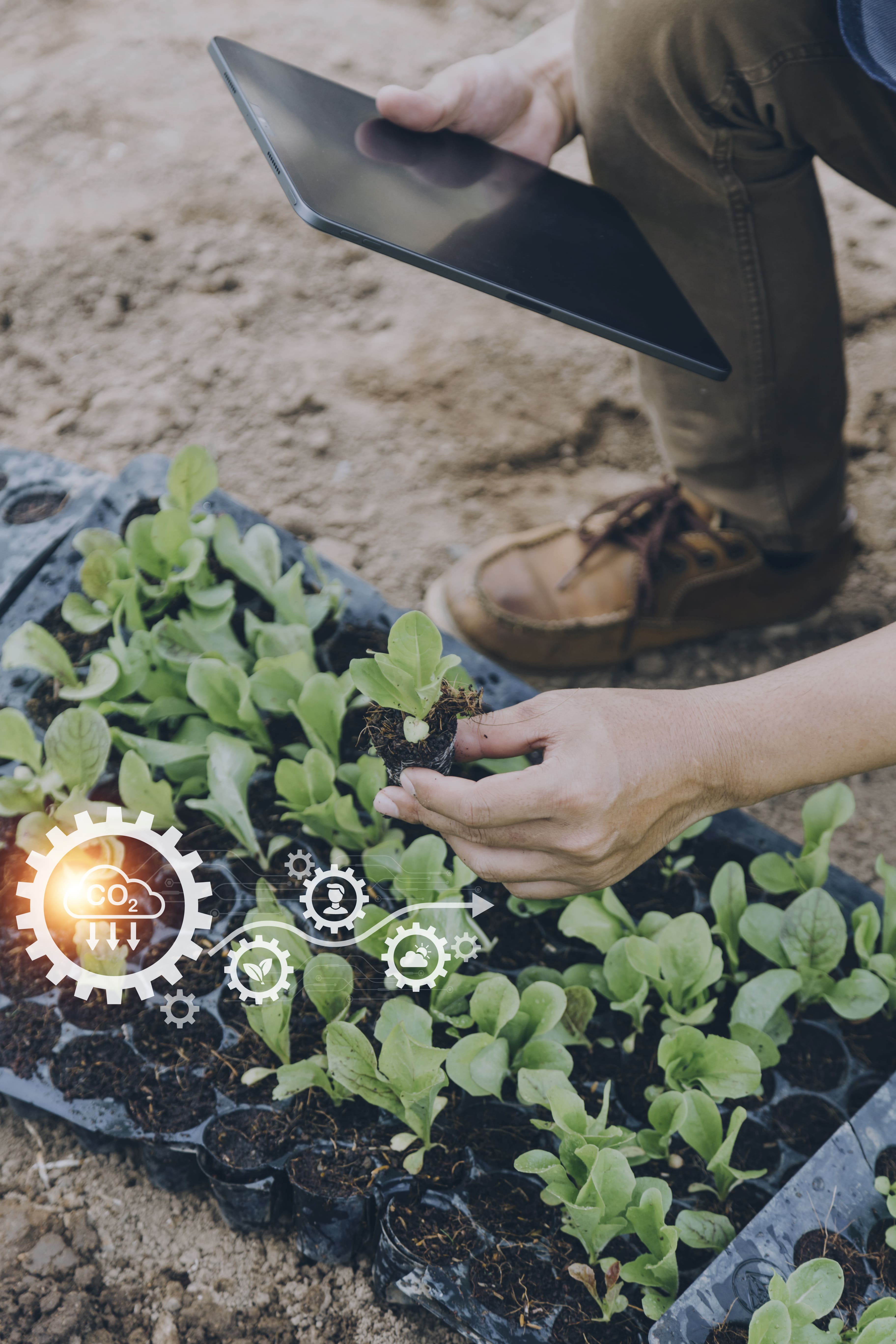
A huge obstacle derailing progress is the absence of sufficient training data that is also well labeled. Researchers have limited options with few datasets available. To handle this challenge, data augmentation is proposed. Data Augmentation is an essential part of enhancing the accuracy of an image classifier. By increasing and diversifying the amount of data, a model is able to handle the problem of overfitting while at the same time generalizing well.
Data Augmentation involves artificially increasing the number of training data using different techniques such as translation, shear, rotation, cutout, among others. Advancement in data augmentation has led to automation of finding the best policies in varying models and datasets. This is different from other methods which create augmented data directly using static augmentation techniques. But in the end, the best solution is for researchers from all over the world to make their raw data easily accessible. When a variety of datasets are available, many solutions will be possible.
PA: How to Implement?
Tools necessary for successful PA implementation include software, hardware and best recommended practices for farming. Global Positioning System (GPS) receivers capture real-time information from GPS satellites. This enables mapping measurements of soil and crops. The second tools are yield monitors. They go hand in hand with GPS receivers by creating yield maps when linked together. Such data is critical for future decision-making. Grid soil sampling as a tool allows sane recommendation of fertilizers within a specified area. The intensity of sampling makes it easy mapping the nutritional need of soil.
Monitoring livestock using AI allows more precise understanding of the animals’ behaviour. The feeding amount, frequency and physical activities can be determined. With real-time data, it is possible to micro-manage livestock without being always physically present.
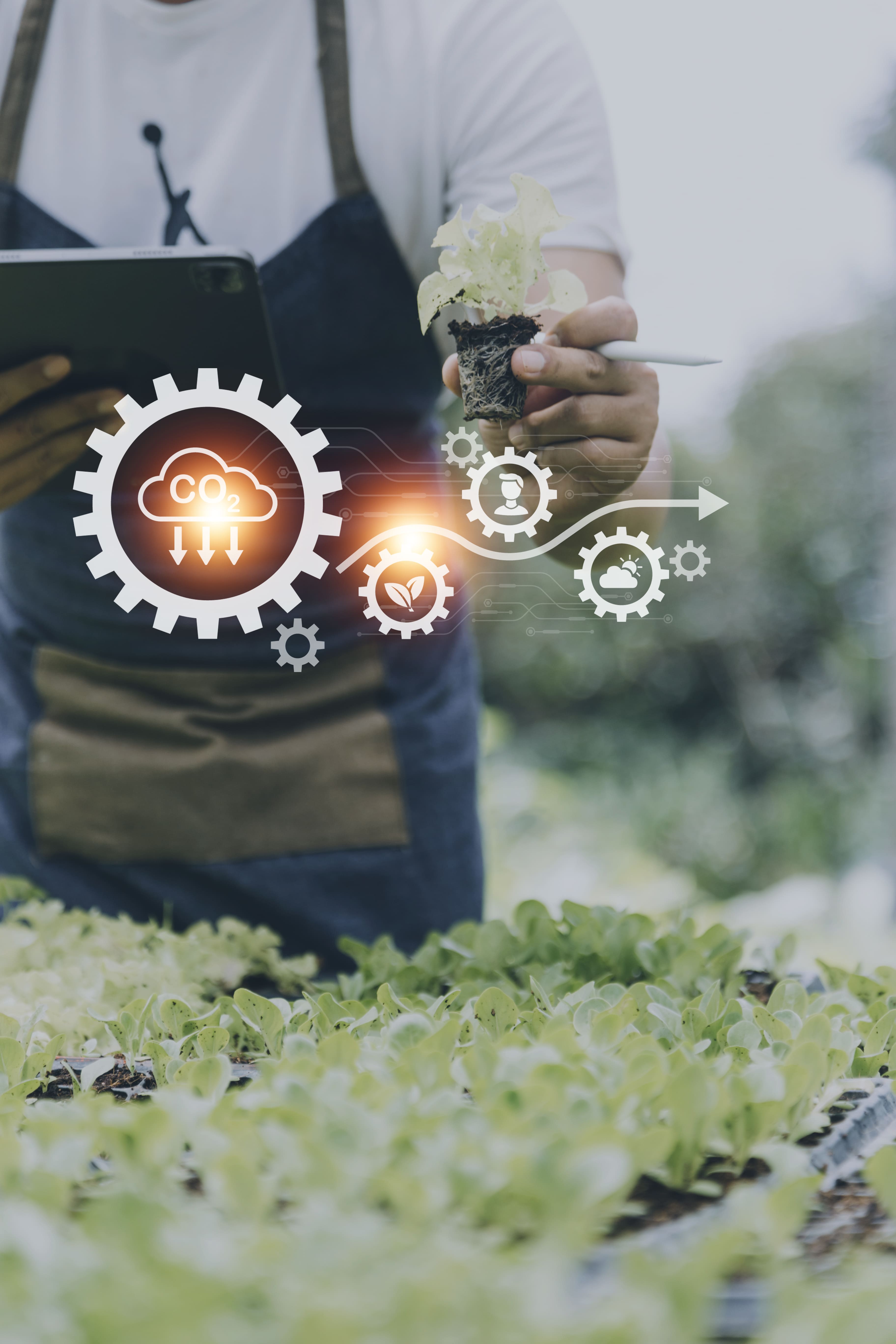
The next tool is Remote Sensing (RS). RS collects data from a distance using a camera held by hand, mounted on drone/aircraft or even satellite. The simplicity of this technique cannot underestimate the amount of data it can provide such as plant stress and in-season variability. The different Vegetation Indexes warrant for deeper plant health analysis. Normalized Difference Vegetation Index (NDVI) is by far the most commonly used index. NDVI measures the productivity of vegetation. This is because it is sensitive to the photosynthetic compounds. Computed using Near Infrared (NIR) and Red (R), the NDVI can ascertain the level of health of vegetation remotely-sensed. Lastly, Information Management as a PA tool helps to make sense of all the data a farmer has been able to collect with time. Relations and hypothesises will be looked at for new information.
In comparison to other fields such vehicle driving or healthcare, the application of AI in agriculture is arguably still at its infancy stage; there exist a huge room for improvement. This could be attributed to a number of factors such as existence of gap between farmers and AI engineers, absence of strong connectivity in farmlands and the farmers lacking time or digital skills to experiment on the possible solutions on their own.
Food demand is always rising, but land for production is growing scarce. The solution is inevitably in improving agriculture. The main challenges facing success in this sector are demographics, scarcity of natural resources, climate change, pests, diseases and food waste. Agriculture is still very much behind in terms of technology adoption. This will help improve efficiency and optimize productivity.
All these go hand in hand with achieving The United Nations Development Programme’s Sustainable Development Goals (SDG). They include No Poverty, Zero Hunger, Good Health and Well-being, Clean Water and Sanitation, lastly, Responsible Consumption and Production.
Information technology is a critical component along with GPS guidance, sensors, robotics, automated hardware, control systems, autonomous vehicles and telematics. The primary goal of PA is profitability, efficiency and sustainability attained through the combination of PA technology and equipment.
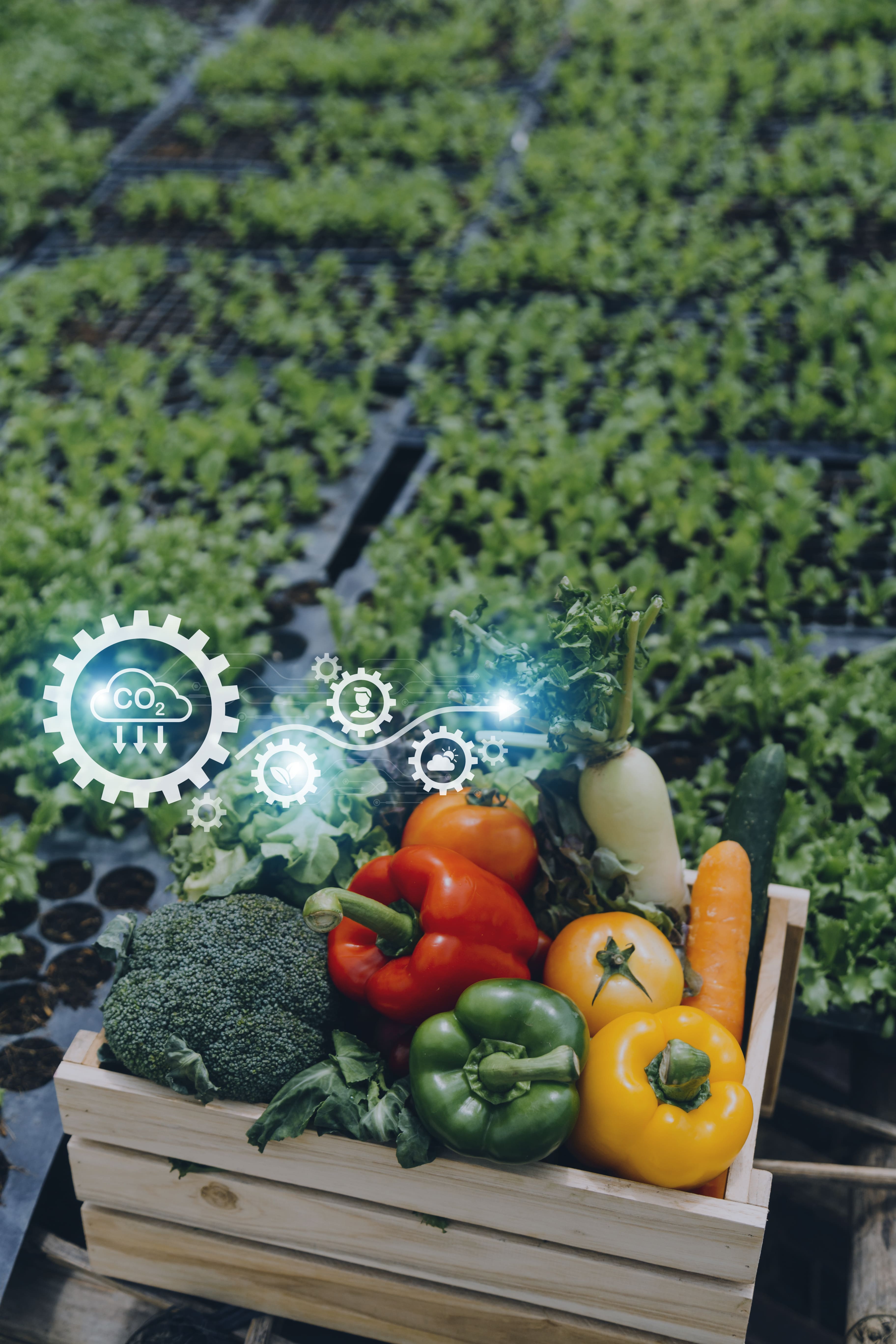
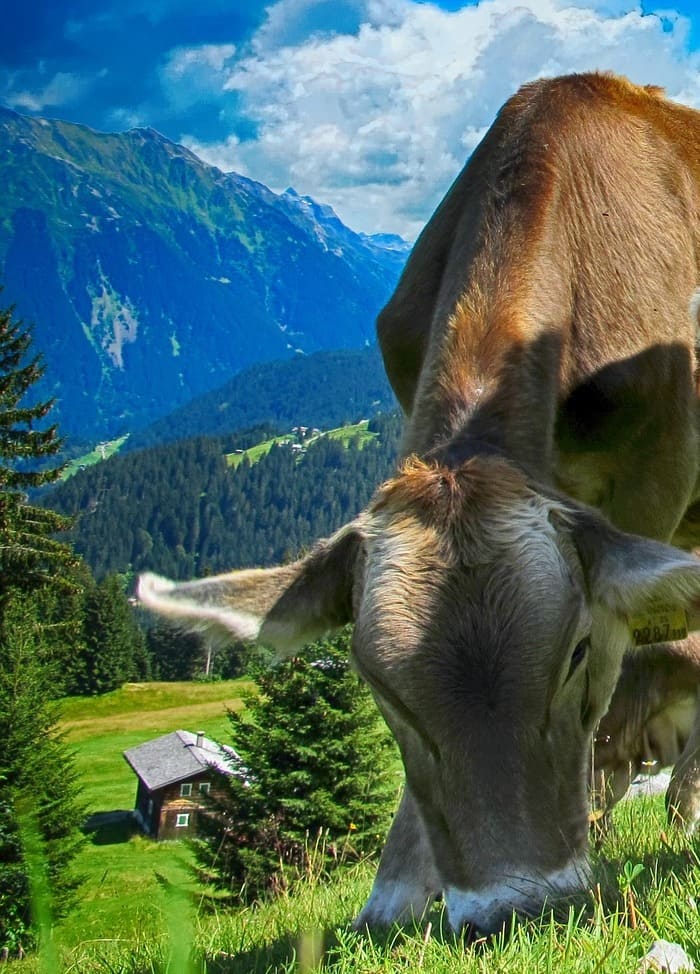
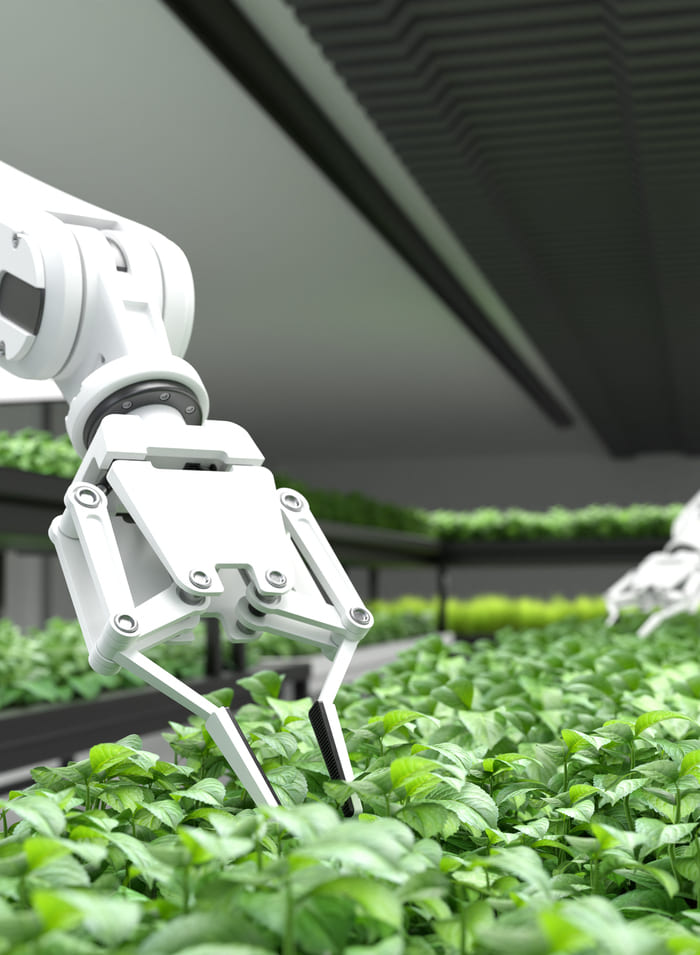
Conclusion
While mankind as a whole society stand to benefit from PA, certain existing constraints limit the optimal gains possible. For starters, the hardware required do not come cheap. Even after purchase, deployment and maintenance still cost a lot. The varying weather conditions also provide a challenge in terms of inconsistent data as well as mechanical distraction. Literacy level in order to properly manage information, hardware and software is still a hurdle.
For as long as agriculture is not the greatest worry of the world, certain challenges which can be easily overcome with simple legislations, such as strong connectivity and different digital standards, will continue to exist.